Just as electricity has spread everywhere and sparked a revolution in society and industry, artificial intelligence (AI) is now everywhere. This invisible hand shapes our world, silently guiding our choices — from video recommendations and the algorithms that drive vehicles and robots — to the important role it plays in drug discovery, diagnoses, and scientific breakthroughs.
Driven by generative AI and its products like ChatGPT, more people are interacting directly with AI. To meet this growing demand, we need specialized computer centers capable of handling the growing needs of both individuals and industries.
AI Factories
Similar to data centers, AI factories comprise a vast network of servers, network devices, and cabling housed in a secure, controlled environment and equipped with an efficient cooling system. However, their function and basic components may vary significantly.
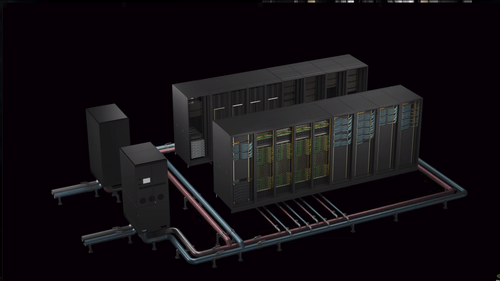
That’s why Nvidia CEO Johnson Huang, speaking at GTC 2024, prefers not to call them data centers. He views them as factories into which raw materials go in, and valuable finished products come out. For example, in power plants, the water goes in, and the electricity comes out.
Likewise, in AI factories, data and electricity go in and tokens come out. These tokens, although intangible, are of great value. They can form different outputs, such as text similar to what Gemini produces, images like those drawn by Midjourney or videos like Sora’s creations. Furthermore, these tokens can be commands to control a robot, a self-driving car, or a drone. They can also generate valuable insights and analyzes that improve work and decision-making in all fields.
While data centers focus on processing, storing, and analyzing data, AI factories are dedicated to training and operating large AI models with high speed and efficiency. Therefore, AI-specific graphical processing units (GPUs), such as Blackwell B200, are the cornerstone and main component in the servers of AI factories.
How does an AI factory create intelligence?
AI factories don’t create intelligence on their own, but rather excel at training and running the AI models that do. They accomplish this through these two main processes:
- Training AI models
After preparing the data and choosing the model architecture, the prepared data is fed into the AI model running on the AI factory’s powerful computing system. Training is an iterative process where the model makes predictions based on the data. These predictions are then compared to the actual data to assess accuracy. This cycle continues until the model achieves a desired level of performance, at which point it can be deployed for real-world AI tasks. This is a complex process that requires significant computational power, time, and energy. AI factories aim to improve all these aspects. Nvidia boasts significant training efficiency improvements with their latest generation of chips, the Blackwell GPUs, the backbone of Nvidia’s AI factories. Previously, training a 1.8 trillion parameter model required 8,000 Hopper GPUs, consuming 15 megawatts of power. The Blackwell architecture, however, achieves the same feat with just four times fewer GPUs (2,000) and a reduction in power consumption of 73% (4 megawatts). - Running AI models
In this process, a trained model is loaded. It then takes the input data and performs calculations to produce the output. This process is called inference. Inference typically requires less computational power compared to training. However, when millions of users access the model, as with LLMs like Gemini and ChatGPT, AI factories with their significant computing power can distribute the workload enabling efficient scaling to handle millions of inference requests simultaneously. For a GPT-3 LLM benchmark with 175 billion parameters, Nvidia claims the Blackwell GB200 delivers a 7x improvement in inference speed compared to the H100.
Who makes AI factories?
AI factories, as envisioned by Nvidia, haven’t been built yet. However, in late 2023, Nvidia announced a collaboration with Foxconn to develop these facilities, likely utilizing Nvidia’s GPUs and software.
In March 2024, Nvidia’s CEO unveiled a digital twin of an AI factory equipped with 32,000 Blackwell B200 GPUs. Notably, he revealed that this factory is being developed specifically for Amazon’s cloud service (AWS). This suggests it could be the first AI factory.
Nvidia is currently a leading provider of AI factories (likely the only one). Companies like AMD and Intel are also likely developing their own solutions, though their current focus might be on creating competitive AI processing units (GPUs) for these factories.
What is the future of AI factories?
We can expect see a lot of them in the near future and new players other than Nvidia will be involved.
A key focus will be on increasing computational power and improving efficiency, particularly in terms of performance and energy savings. This involves optimizing the performance of graphic processing units (GPUs) and improving network devices to accelerate data transfer between them.
The development of AI factories hinges on a crucial aspect: their users. Researchers and developers of AI models rely on these factories to build larger, more complex models that tackle intricate tasks across various industries and benefit countless individuals. This, in turn, fuels the need for even bigger and more efficient factories, creating a continuous cycle of development.
Thus, AI factories are fueling the development of increasingly complex AI models, and vice versa. These advanced models, in turn, demand even more efficient AI factories.